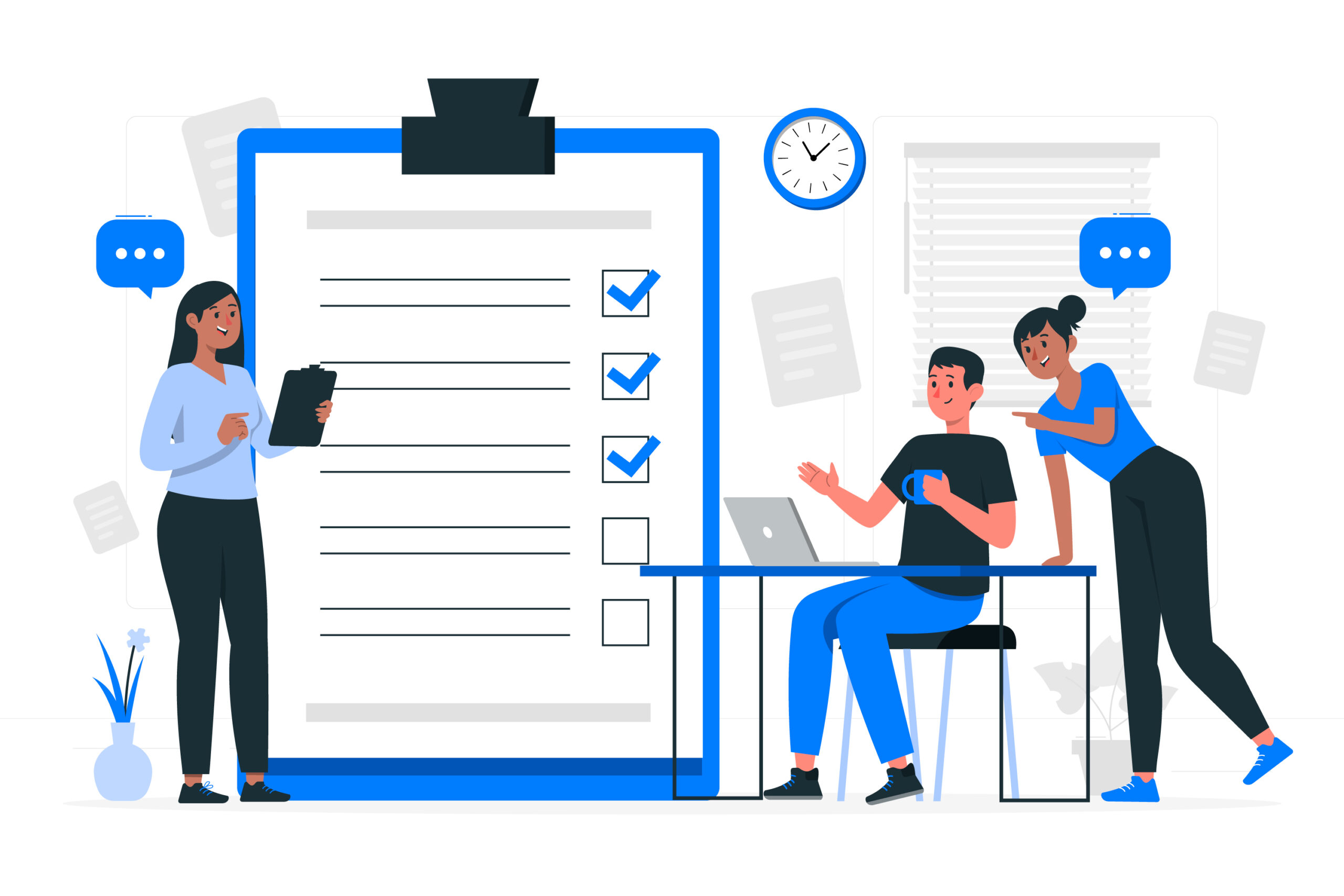
In the world of facility management, predictive maintenance has emerged as a powerful strategy for optimizing equipment performance, reducing downtime, and cutting maintenance costs. By using data-driven insights to anticipate equipment failures before they occur, predictive maintenance promises to transform how facilities are managed. However, many facility managers face significant challenges when implementing predictive maintenance, especially when dealing with limited data.
The Promise of Predictive Maintenance
Predictive maintenance relies on data collected from various sensors and systems within a facility to monitor equipment health. By analyzing this data, facility managers can identify patterns and trends that indicate potential issues before they lead to equipment failure. This proactive approach allows for maintenance to be performed only when necessary, reducing unnecessary repairs, extending the life of equipment, and preventing costly downtime.
However, the effectiveness of predictive maintenance is heavily dependent on the quality and quantity of data available. When data is limited or incomplete, the ability to accurately predict equipment failures is compromised, leading to several implementation challenges.
Challenges of Implementing Predictive Maintenance with Limited Data
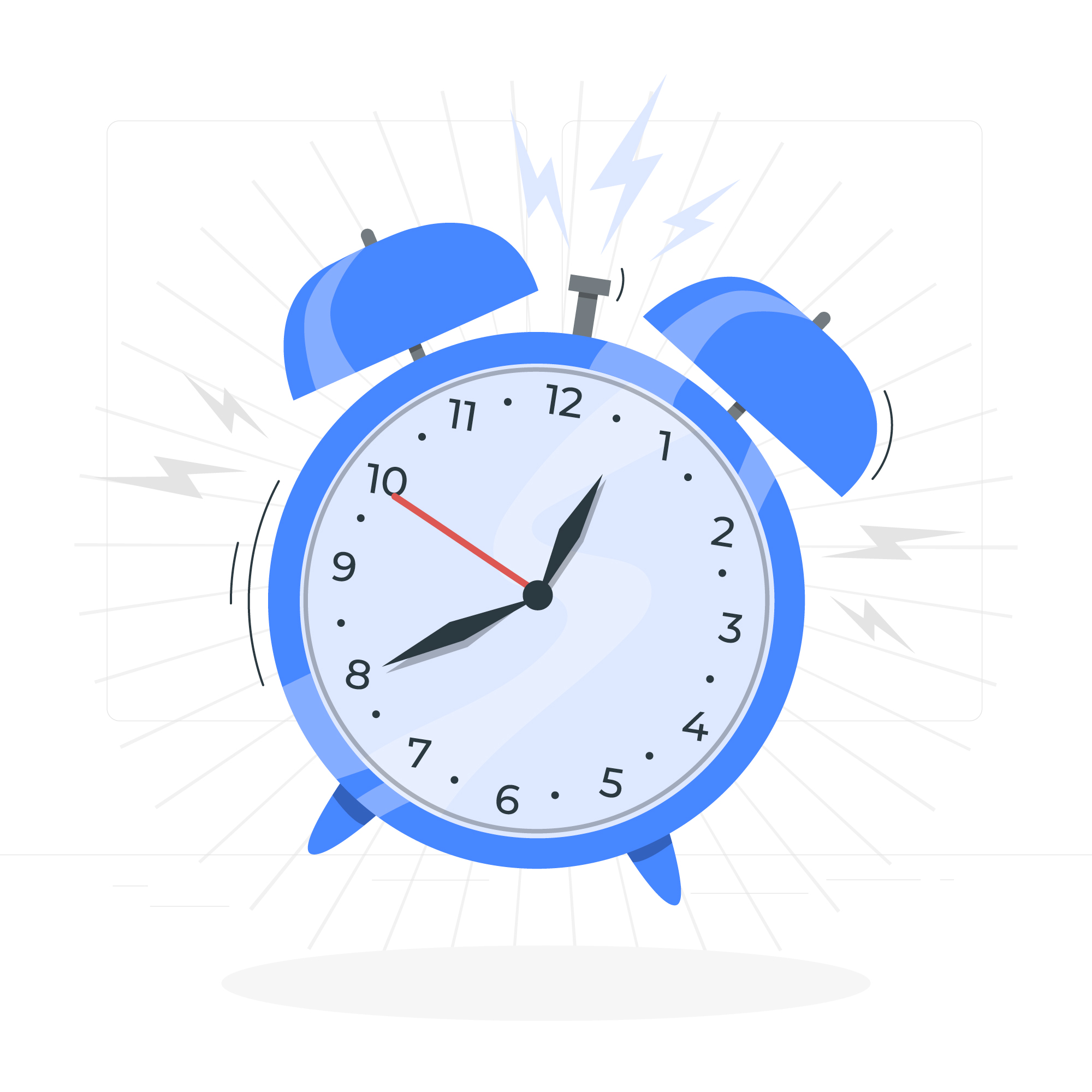
1. Inaccurate Predictions
One of the most significant challenges facility managers face with limited data is the risk of inaccurate predictions. Predictive maintenance algorithms rely on historical data to identify patterns that precede equipment failures. When the data is sparse or does not cover a long enough period, the algorithms may struggle to recognize these patterns, leading to false positives or negatives. Inaccurate predictions can result in unnecessary maintenance activities, or worse, missed opportunities to prevent equipment failure.
2. Integration with Existing Systems
Many facilities have legacy systems that were not designed with predictive maintenance in mind. Integrating these systems with modern predictive maintenance tools can be challenging, especially when data is limited. Legacy systems may lack the necessary sensors or data collection capabilities, making it difficult to gather the information needed for accurate predictions. This integration challenge can slow down the implementation process and increase costs.
3. Data Quality Issues
Even when data is available, it may not always be of high quality. Data quality issues, such as inconsistencies, missing values, or inaccurate sensor readings, can severely impact the effectiveness of predictive maintenance. Facility managers need to invest time and resources in cleaning and validating data before it can be used for predictive purposes. However, with limited data, there may not be enough information to identify and correct these issues, leading to unreliable predictions.
4. Lack of Expertise
Implementing predictive maintenance requires specialized knowledge in data analytics, machine learning, and facility management. Many facility managers may lack the expertise needed to effectively leverage predictive maintenance tools, especially when working with limited data. This knowledge gap can lead to suboptimal implementation and reduced benefits from the predictive maintenance program.
5. High Initial Costs
The initial costs of implementing predictive maintenance can be high, particularly when additional sensors, data storage, and analytics tools are required. For facilities with limited data, these costs can be even higher, as additional investments may be needed to enhance data collection capabilities. Without sufficient data to demonstrate the potential ROI, it can be challenging to justify these upfront costs to stakeholders.
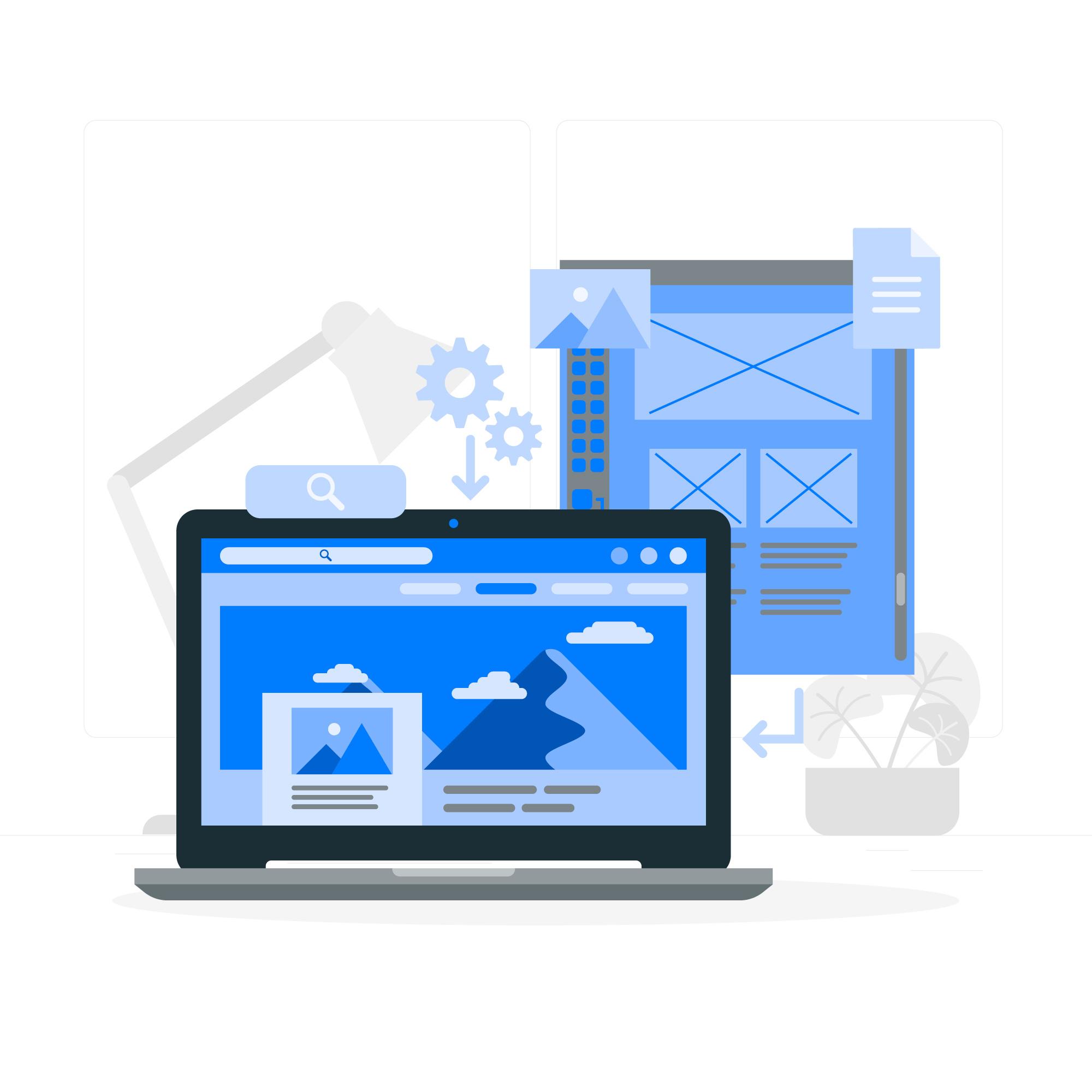
How SwevenBPM Can Help Overcome These Challenges
SwevenBPM offers a comprehensive solution to help facility managers overcome the challenges of implementing predictive maintenance, even with limited data. Here’s how:
1. Enhanced Data Collection and Integration
SwevenBPM is designed to integrate seamlessly with existing Building Management Systems (BMS) and legacy systems, enabling facility managers to leverage the data they already have. Additionally, SwevenBPM supports the deployment of additional sensors and data collection devices, helping to fill in gaps where data is limited. By aggregating data from multiple sources, SwevenBPM provides a more complete picture of equipment health, improving the accuracy of predictions.
2. Advanced Data Analytics and Machine Learning
SwevenBPM employs advanced data analytics and machine learning algorithms that are specifically designed to work with limited data sets. These algorithms can identify patterns and trends even in sparse data, providing more accurate predictions than traditional methods. SwevenBPM also includes data validation and cleaning tools to ensure that the data being used for predictive maintenance is of the highest quality.
3. User-Friendly Interface and Training
Understanding the need for specialized expertise, SwevenBPM offers a user-friendly interface that makes it easier for facility managers to implement and manage predictive maintenance programs. The platform provides step-by-step guidance and built-in tutorials to help users make the most of the tools available. Additionally, SwevenBPM offers training programs to bridge the knowledge gap, ensuring that facility managers have the skills they need to succeed.
4. Cost-Benefit Analysis and ROI Calculation
SwevenBPM includes tools for conducting cost-benefit analysis and calculating the ROI of predictive maintenance initiatives. This allows facility managers to demonstrate the potential savings and benefits of predictive maintenance to stakeholders, even when starting with limited data. By providing a clear financial justification, SwevenBPM helps secure the necessary investment for implementation.
5. Scalability and Flexibility
SwevenBPM is a scalable solution that can grow with your facility’s needs. As more data becomes available, the platform’s predictive maintenance capabilities will continue to improve, providing even greater accuracy and reliability. This flexibility ensures that your investment in SwevenBPM will continue to deliver value over time, regardless of the initial data limitations.
Conclusion: Turning Limited Data into a Strategic Advantage
While limited data presents a significant challenge for implementing predictive maintenance, it doesn’t have to be a roadblock. With the right tools and strategies, facility managers can overcome these challenges and unlock the full potential of predictive maintenance. SwevenBPM offers a comprehensive, data-driven solution that helps facility managers make the most of the data they have, providing accurate predictions, reducing downtime, and optimizing maintenance costs.
By leveraging SwevenBPM’s advanced analytics, user-friendly interface, and integration capabilities, facility managers can turn limited data into a strategic advantage, ensuring the success of their predictive maintenance programs. Don’t let data limitations hold you back—embrace the power of predictive maintenance with SwevenBPM and take your facility management to the next level.